Powering Predictability: How PrimeNeuro Principals Revolutionized Maintenance Analytics for GE Aircraft Engines
At PrimeNeuro, we pride ourselves on solving complex challenges with tailored AI-driven solutions. When General Electric Aircraft Engines, a global leader in aviation, sought to optimize their Maintenance Cost Per Hour (MCPH) program, they approached us with a clear mission: enhance predictability in maintenance costs while maximizing operational efficiency for their clients.
The MCPH program is central to GE Engine Services, offering commercial and corporate aircraft operators a fixed rate per engine flight hour. However, GE faced increasing complexity in forecasting long-term costs, particularly as newer engine models and enhanced performance upgrades emerged. The challenge was compounded by the need to analyze vast amounts of maintenance data across different engine models, such as the CF34, CFM56, and CF6 series, while meeting the varying demands of diverse operators.
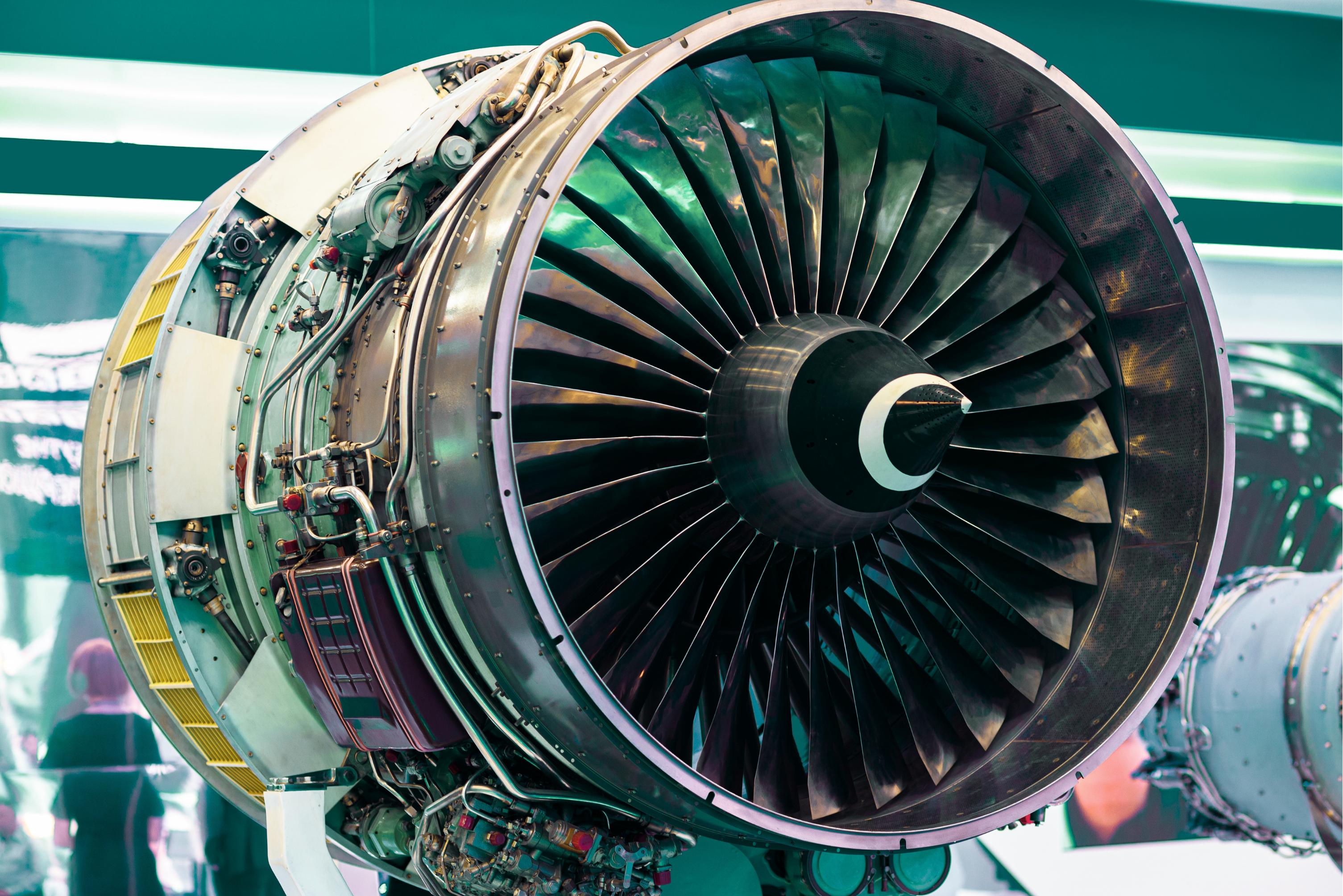
Understanding these challenges, we partnered with GE to design an AI-powered predictive analytics solution tailored specifically to the MCPH program. Leveraging machine learning algorithms, we developed a platform that analyzed historical maintenance data, operational trends, and real-time engine performance metrics. This platform provided actionable insights, enabling GE to forecast maintenance needs and costs with unprecedented accuracy.
The solution integrated seamlessly with GE’s existing systems and included a dynamic dashboard for real-time monitoring and reporting. Our predictive models accounted for variables such as engine usage patterns, regional environmental factors, and the impact of performance-enhancing upgrades, like the Enhanced Performance kit for CFM56 engines.
By capturing these nuances, the tool provided GE with the granularity needed to structure MCPH agreements that aligned perfectly with each client’s operational realities.
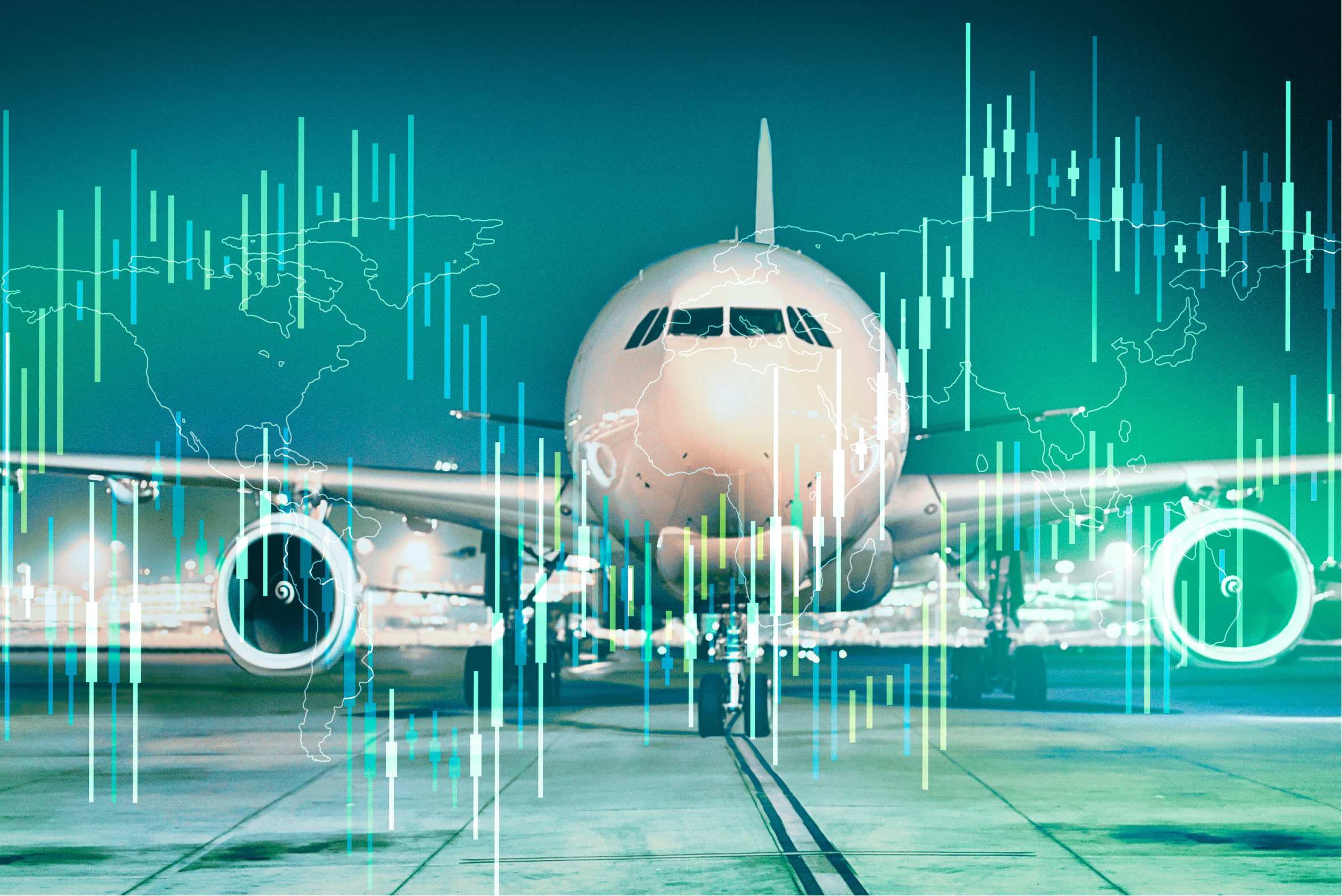
The results were transformative. Within six months of implementation, GE reported a 30% improvement in cost prediction accuracy for the MCPH program. This enhanced precision allowed their clients to budget with greater confidence, significantly reducing unexpected expenses. Furthermore, GE’s internal teams benefited from streamlined processes, cutting the time required to generate customized MCPH agreements by 40%. The predictive insights also supported strategic decision-making, enabling GE to identify opportunities for performance upgrades that extended engine lifespans and reduced overall maintenance costs.
Our work with GE demonstrates the power of harnessing AI to solve industry-specific challenges. By marrying advanced technology with a deep understanding of aviation maintenance, we not only elevated the MCPH program but also set a new benchmark for efficiency and reliability in the field.